The keys to effective AI regulation are hiding in plain sight.
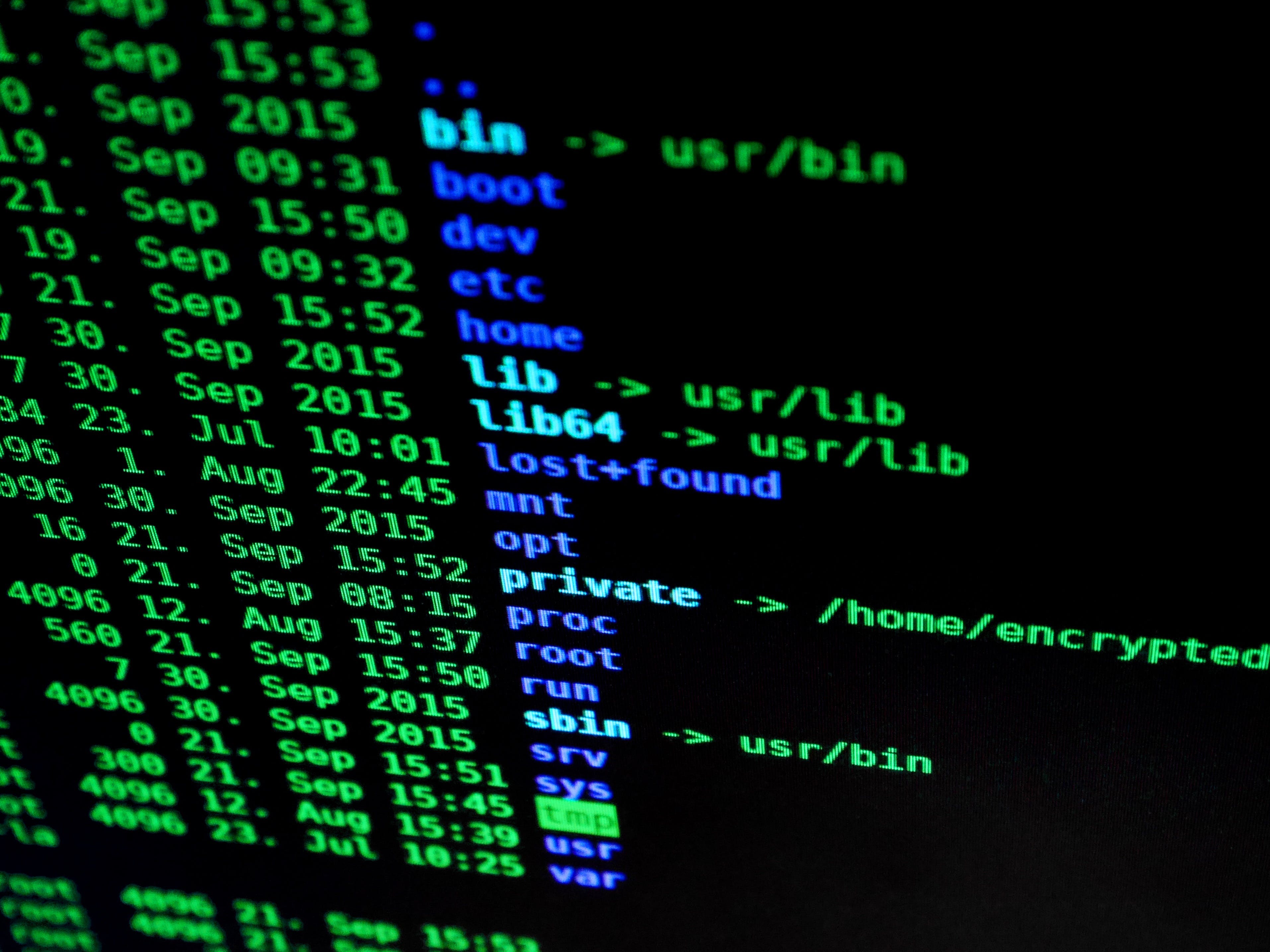
The November 2023 implosion of OpenAI—the creator of the viral artificial intelligence (AI) chatbot ChatGPT—has intensified debates over how AI should be governed. Just weeks before Sam Altman was fired and then quickly rehired as the company’s CEO, the White House had announced new safety standards for developers of generative AI, the technology on which ChatGPT is based. Discussions about generative AI’s risks also dominated the United Kingdom’s first high-level summit on AI safety. And disagreement about what constraints should be placed on the rapidly developing technology nearly derailed the European Union’s long-awaited regulation on AI.
This preoccupation with generative AI is overshadowing other forms of algorithmic decision-making that are already deeply embedded in society and the lessons they offer on regulating emerging technology. Some of the algorithms that attract the least attention are capable of inflicting the most harm—for example, algorithms that are woven into the fabric of government services and dictate whether people can afford food, housing, and health care.
In the U.K., where Prime Minister Rishi Sunak recently warned that “humanity could lose control of AI completely,” the government has ceded control of the country’s social assistance system to algorithms that often shrink people’s benefits in unpredictable ways. The system, known as Universal Credit, was rolled out in 2013 to improve administrative efficiency and save costs, primarily through automating benefits calculations and maximizing digital “self-service” by benefit claimants.
The ballooning costs of implementing Universal Credit have raised doubts about the government’s promise of cost savings. This largely automated system is also making the process of claiming benefits and appealing errors more inflexible and burdensome for people already struggling with poverty.
U.K. civil society groups as well as my organization, Human Rights Watch, have found that the system relies on a means-testing algorithm that is prone to miscalculating people’s income and underestimating how much cash support they need. Families that bear the brunt of the algorithm’s faulty design are going hungry, falling behind on rent, and becoming saddled with debt. The human toll of automation’s failures appears to be lost on Sunak, however, who has vowed that his government will not “rush to regulate” AI.
Sunak has also hailed AI as the “answer” to “clamping down on benefit fraudsters,” but the experience of other European countries tells a different story. Across the continent, governments are turning to elaborate AI systems to detect whether people are defrauding the state, motivated by calls to reduce public spending as well as politicized narratives that benefits fraud is spiraling out of control. These systems tap into vast pools of personal and sensitive data, attempting to surface signs of fraud from people’s national and ethnic origin, the languages they speak, and their family and employment histories, housing records, debt reports, and even romantic relationships.
These surveillance machines have not yielded the results governments have hoped for. Investigative leads flagged by Denmark’s fraud detection algorithm make up only 13 percent of the cases that Copenhagen authorities investigate. An algorithm that the Spanish government procured to assess whether workers are falsely claiming sick leave benefits suffers from high error rates—medical inspectors who are supposed to use it to investigate claims have questioned whether it is even needed at all.
More importantly, these technologies are excluding people from essential support and singling them out for investigation based on stereotypes about poverty and other discriminatory criteria. In France, a recent Le Monde investigation found that the country’s social security agency relies on a risk-scoring algorithm that is more likely to trigger audits of rent-burdened households, single parents, people with disabilities, and informal workers. In 2021, the Dutch government was forced to resign after revelations that tax authorities had wrongly accused as many as 26,000 parents of committing child benefits fraud, based on a flawed algorithm that disproportionately flagged low-income families and ethnic minorities as fraud risks.
EU policymakers struck a deal on Dec. 8, 2023, to pass the Artificial Intelligence Act, but French President Emmanuel Macron has since voiced concern that the regulation could stymie innovation. Even if the deal holds, it is doubtful that the regulation will impose meaningful safeguards against discriminatory fraud detection systems, let alone curb their spread. Previous drafts of the regulation had proposed a vague ban on “general purpose” social scoring, invoking the specter of a dystopian future in which people’s lives are reduced to a single social score that controls whether they can board a plane, take out a loan, or get a job. This fails to capture how fraud risk scoring technologies currently work, which serve a more limited purpose but are still capable of depriving people of lifesaving support and discriminating against vulnerable populations.
Harmful deployments of AI are also piling up in the United States, and legislative gridlock is stalling much-needed action. Many states, for example, are relying on facial recognition to verify applications for unemployment benefits, despite widespread reports that the technology has delayed or wrongly denied people access to support. More than two dozen states have also deployed algorithms that are linked to irrational cuts in people’s home care hours under a Medicaid program that provides support for independent living.
In 2017, Legal Aid of Arkansas successfully challenged the state’s Medicaid algorithm, which replaced assessments of home care needs by nurses. The algorithm arbitrarily slashed home care hours allotted to many older people and people with disabilities, forcing them to forgo meals, baths, and other basic tasks they need help with.
Kevin De Liban, the Legal Aid lawyer who brought the lawsuit, told me that the switch to algorithmic assessments was bound to create hardship because many people did not have access to adequate support to begin with. When Arkansas rolled out the algorithm, it also reduced the weekly cap on home care support from 56 to 46 hours. “People with disabilities would struggle to live independently under the best-case scenario,” said De Liban. “When the algorithm allocates even less hours than that, the situation becomes totally intolerable and results in immense human suffering.”
Congress has proposed a flurry of bills to regulate these technologies, but the most concrete measure so far has come from the White House. Its recent executive order directs generative AI developers to submit safety test results and federal agencies to establish guidelines on how they use AI. In particular, federal agencies overseeing Medicaid, food assistance, and other benefits programs are required to draw up guidance on automating these programs in a manner that ensures transparency, due process and “equitable outcomes.”
What the order does not do, however, is impose red lines against applications of the technology that seem to be prone to abuse, such as facial recognition, predictive policing, and algorithmic risk assessments in criminal sentencing. Executive orders are also no substitute for legislation: Only Congress can grant federal authorities new powers and funding to regulate the tech industry, and only Congress can impose new restrictions on how states spend federal dollars on AI systems.
AI’s harm to public services offers a road map on how to meaningfully regulate the technology, and the costs of falling short. Perhaps the most critical learning is the need to set up no-go zones: bans on applications of AI that pose an unacceptable risk to people’s rights. As EU policymakers finalize the language of the AI Act in the coming weeks, they have one last opportunity to tailor the regulation’s social scoring ban to AI-facilitated abuses in public services. A handful of U.S. cities have banned their government agencies from using facial recognition, but they remain the exception despite mounting evidence of the technology’s role in wrongful arrests.
These bans should also be considered when the risks posed by AI cannot be sufficiently mitigated through due diligence, human oversight, and other safeguards. Arkansas’s disastrous rollout of an algorithm to allocate in-home care is a case in point. In response to legal challenges, the state established a new system that continued to rely on an algorithm to evaluate eligibility but required health department officials to check its assessments and modify them as needed. But arbitrary cuts to people’s home care hours persisted: A 71-year-old woman with disabilities who sued the state for cutting her hours said she went hungry, sat in urine-soaked clothing, and missed medical appointments as a result. Despite additional safeguards, the overriding objective of the algorithm-assisted system remained the same: to reduce costs by cutting support.
For AI systems that raise manageable risks, meaningful regulation will require safeguards that prompt scrutiny of the policy choices that shape how these systems are designed and implemented. Well-intentioned processes to reduce algorithmic bias or technical errors can distract from the need to tackle the structural drivers of discrimination and injustice. The catastrophic effects of the U.K.’s Universal Credit and Arkansas’s Medicaid algorithm are as much a consequence of austerity-driven cuts to the benefits system as they are of overreliance on technology. Spain’s sick leave monitoring algorithm, medical inspectors say, is hardly a solution to long-standing staff shortages they are experiencing.
De Liban, the Legal Aid lawyer, told me that trying to fix the algorithm sometimes distracts from the need to fix the underlying policy problem. Algorithmic decision-making is predisposed to inflict harm if it is introduced to triage support in a benefits system that is chronically underfunded and set up to treat beneficiaries as suspects rather than rights-holders. By contrast, benefits automation is more likely to promote transparency, due process, and “equitable outcomes” if it is rooted in long-term investments to increase benefit levels, simplify enrollment, and improve working conditions for caregivers and caseworkers.
Policymakers should not let hype about generative AI or speculation about its existential risks distract them from the urgent task of addressing the harms of AI systems that are already among us. Failing to heed lessons from AI’s past and present will doom us to repeat the same mistakes.
– Amos Toh is a senior tech and human rights researcher at Human Rights Watch., Published courtesy of Lawfare.