Shifting the focus of AI liability from the systems to the builders.
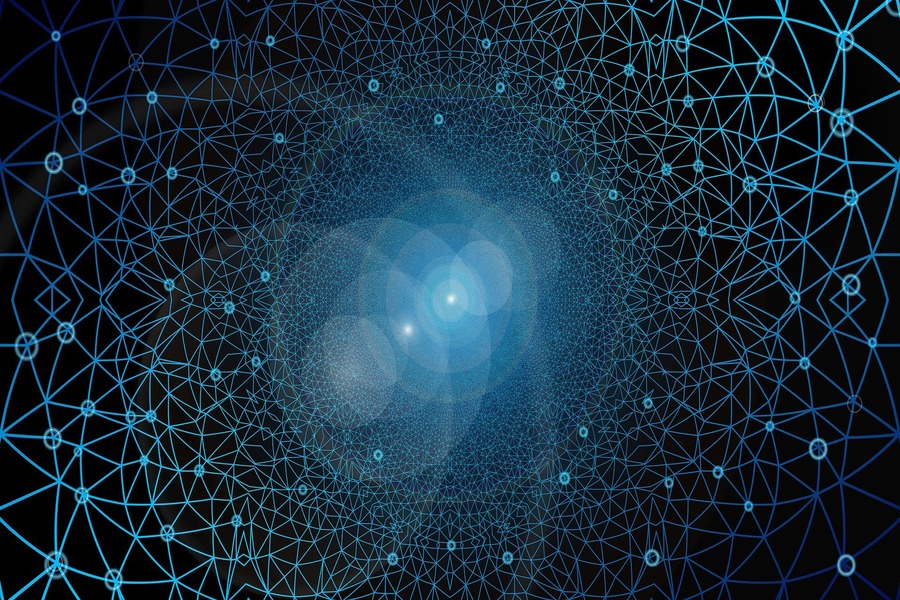
Editor’s note: This essay is part of a series on liability in the AI ecosystem, from Lawfare and the Georgetown Institute for Technology Law and Policy.
To date, most popular approaches to AI safety and accountability have focused on the technological characteristics and risks of AI systems, while averting attention from the workers behind the curtain responsible for designing, implementing, testing, and maintaining such systems. Efforts like the EU AI Act epitomize this approach, in that they condition regulatory oversight based on technical attributes such as the amount of compute used for training or tuning an AI model. Likewise, the products liability framework for AI points the finger at unsafe product features and minimizes the conduct of human decision-makers. Other proposals such as strict liability or analogies of “sentient” AI to children or wild animals are similarly avoidant of engaging with the human processes by which AI is made. This technological focus allows AI engineers to dissociate themselves from the harms they impose on others.
I have previously argued that a negligence-based approach is needed because it directs legal scrutiny on the actual persons responsible for creating and managing AI systems. A step in that direction is found in California’s AI safety bill, which specifies that AI developers shall articulate and implement protocols that embody the “developer’s duty to take reasonable care to avoid producing a covered model or covered model derivative that poses an unreasonable risk of causing or materially enabling a critical harm” (emphasis added). Although tech leaders have opposed California’s bill, courts don’t need to wait for legislation to allow negligence claims against AI developers. But how would negligence work in the AI context, and what downstream effects should AI developers anticipate?
Basics of Negligence Law
Negligence is a fault-based theory of tort law that obligates people to act with proper care. If one’s failure to exercise due care causes harm to another individual, then one can be found at fault and the court can order payment of compensatory and punitive damages. Plaintiff-victims need to prove their claim by a preponderance of the evidence (i.e., more than 50 percent likelihood of being true). Typically, legal scholars parse the negligence claim into four (or five) elements: duty, breach, factual and proximate cause, and injury. Because negligence is a creature of state common law, it can vary state-by-state in how those elements are stated, interpreted, and applied in individual cases. That said, the essential gist of negligence remains the same: undue carelessness.
In the AI context, a liability regime based on negligence would examine whether creators of AI-based systems have been careful enough in the design, testing, deployment, and maintenance of those systems. By extension, a negligence theory against users of AI systems would hold them at fault for their careless use of pretrained systems.
Breach of Due Care
How much care is “good enough” to avoid liability? The usual default expectation is “reasonable care,” or what the “reasonably prudent person” would have done. Ordinarily a jury of peers decides what is reasonable. Alternatively, for some well-defined situations—for example, traffic safety laws—a legislature or agency can set forth statutory violations that serve as per se proof of unreasonableness, or “negligence per se.” And in special circumstances, a different standard of care can be substituted altogether, such as utmost care, customary care, or gross negligence (failure to use even slight care).
The reasonableness standard is an objective standard that holds all similarly situated persons to the same expectation of care. It represents a modern shift from thinking about due care merely as ordinary care (how people usually behave) to the normative lens of reasonable care (how people should behave). For example, all drivers are judged the same whether they are professional race car drivers or newly licensed teenagers. Likewise, every AI modeler—from unpaid hobbyist to paid expert—would be expected to be familiar with all concepts that the reasonably prudent AI modeler would know. Pleading ignorance of a commonly used technique would be ineffective as an excuse. Nor is it sufficient to merely comply with industry standards, if the entire industry lags behind acceptable levels of precaution. At the same time, the reasonable AI modeler would not be expected to incorporate cutting-edge research, especially where it is not well established in practice.
Reasonableness is also a flexible standard that takes into account contextual information about the knowledge and skills of a similarly situated class of people. Thus, an AI modeler might be expected to have the equivalent of a doctorate in machine learning, while a data analyst who cleans training data might be compared only to a high school or college graduate. Other contextual factors influencing determinations of reasonable care might include the type of AI model or system, the application domain, and the market conditions. For example, reasonable care for autonomous vehicle systems might look substantially different from reasonable care for large language models, and so on.
Although reasonableness works well as a standard of care when there is shared consensus about community norms, it functions less well in contexts where opinions are deeply fractured and contested. For example, long-standing efforts to define “reasonable” software development practices have proved elusive, with sharp divisions among factions and little evidence to show that any one approach is obviously safer than another.
Whether AI developers will find common methodological ground or will devolve into guilded sects remains to be seen. Recently, the White House ordered federal agencies to develop guidelines, standards, and best practices for AI safety and security. If such standards are developed in a useful manner, they could aid in determining unreasonableness, or even form the basis for claims based on negligence per se. Conversely, compliance with such standards could offer persuasive evidence, though not automatic proof, of reasonableness.
Some reasons for optimism toward consensus standards include the fact that the machine learning techniques being used with commercial success are relatively homogeneous and that the AI developer community remains relatively small with significant skill-based and resource-based barriers to entry. All commercial AI systems are built using data-driven machine learning methods. The most capable AI models use deep neural networks and a narrow set of architectural and hyperparameter choices that have been forged and reforged over time through guesswork and empirical study. Those technological and market constraints indicate the likelihood that there are broad swaths of agreement on standard methods and practices in the field.
At the same time, early forays into creating AI standards have been vague and diffuse, centering on sociotechnical values that are readily contested and unlikely to produce consensus. A representative example is the AI Risk Management Framework issued by the National Institute of Standards and Technology. The framework enumerates many broad principles—including validity, safety, resilience, accountability, explainability, privacy, and fairness—but notes that choosing metrics and threshold values depends on “human judgment.” Worse, the framework notes a “current lack of consensus on robust and verifiable measurement methods,” meaning that the uncertainties and disagreements run deeper than mere risk tolerance.
One area where the law substitutes a different standard of care is in “professional” contexts such as medicine and law, where it is common to encounter strongly differing opinions about best practices. Accordingly, physicians and attorneys are held to a “customary care” standard, which imposes liability only when an individual’s conduct deviates from an established custom of practice. The key doctrinal difference is that where there are multiple schools of thought, adherence to a minority tradition (such as alternative medicine) is accepted as meeting due care, even if practitioners of other traditions would vehemently disagree. The customary standard of care determines reasonableness based on actual practices in the field, as opposed to normative claims about what “should” happen, precisely because the field lacks consensus on reasonable practices.
Arguably, the customary care standard is a better fit for AI liability, because practices are still evolving rapidly and a single reasonableness standard would be too difficult to distill across heterogeneous entities and application domains. Allowing breathing room for different approaches to flourish may be more appropriate than predetermining which ones are or are not reasonable. Importantly, although the customary care standard defers to professional experts, it remains a negligence action that differs from self-regulation or regulatory forbearance in at least two respects. First, it prompts AI experts to attest in court what their understanding of the industry custom is, which promotes transparency regarding AI best practices. Second, it facilitates liability for obviously unacceptable practices, which are evident even without expert opinion. By analogy, in the cybersecurity context, entities like the Federal Trade Commission and the Center for Internet Security have begun to compile lists of worst cybersecurity practices. A similar effort is needed for AI development practices. In these ways, the customary care standard enables a modicum of legal oversight despite the uncertainties and fast-moving nature of the technological expertise.
Another possible standard is “fiduciary care,” which has been touted by prominent scholars who urge that big data custodians—including AI developers—who solicit our trust should be held accountable as information fiduciaries. Here, the usual duty of care is modified by adding a duty of loyalty, which involves placing the data subject’s interests ahead of the data custodian’s own interests. Other commentators have questioned whether such an arrangement is feasible when there are divided loyalties to conflicting stakeholders such as users versus corporate shareholders. Moreover, it is unclear how a duty of loyalty would actually alter the job performance of AI developers. Would they be required to collect less data? Train and test the AI models more rigorously or ethically than before? Customize safety features for individual users?
Or perhaps AI developers should be held instead to a duty of “utmost care,” by analogy to innkeepers and common carriers. This heightened duty obligates those carriers to exercise especially high care to ensure the safety of their clientele. A parallel debate asks whether internet service providers and internet platforms should be construed as common carriers for non-tort purposes. If that logic extends to AI-based platforms in the regulatory context, then the common carrier designation ought to apply in tort analysis as well. Yet, while it sounds ideal in principle to command AI developers to exercise the best possible care, the specific marginal changes to the baseline duty remain as hazy as before. Many courts and commentators disagree that degrees of care can be meaningfully distinguished. Furthermore, if the reasonableness standard cannot be easily conceptualized, then a reasonableness-plus-more standard is equally vague. Without clear limitations on scope, the rhetoric of utmost care threatens to tip over into a pure strict liability regime, which would spur liability that is both excessive and untethered from notions of fairness rooted in human fault.
Limitations on Negligence Liability
While negligence liability is an important backstop that looms over social behavior, it is a limited remedy that is not meant to repair all social harms. A host of doctrinal and statutory bars mark the boundaries where negligence law stops and allows the cost of accidents to go uncompensated.
Injury
To state a negligence cause of action, there must be a qualifying injury. Risky conduct alone is not actionable, even if it is reprehensible. For example, a driver who runs a red light without causing injury may receive a traffic citation but will not be found negligent. There must be an actual, concrete injury for the law to recognize the tort claim.
The paradigmatic injury is bodily harm or property damage. Economic losses such as lost wages or medical bills are also compensable as long as they arise out of the physical injury. Narrow categories of informational harms such as defamation and failure to warn are also eligible injuries. Some scholars have observed that the law’s focus on physical injury reflects a historical bias favoring claims brought by men over ones brought by women. For example, emotional distress or psychic harm is often insufficient standing alone unless tied to physical impacts or physical manifestations of injury—although those restrictions have become somewhat looser in modern times.
By contrast, courts often dismiss cases when they claim only pure economic loss such as lost profits or lost goodwill. Unlike physical injuries, pure economic injuries are diffuse and intangible, which makes the dollar-cost amount harder to quantify and threatens to open the floodgates to boundless liability. As a result, courts often reason that such disputes should be resolved (if at all) through contract law, where the parties’ mutual agreement on risk allocation can be set forth in a document. However, a minority of courts allow recovery for pure economic loss if it can be limited in an identifiable way. Furthermore, the economic loss rule does not apply to professional malpractice cases. Data breach cases have also emerged as a notable exception to the economic loss rule. That said, many scholars have lamented that claims of privacy harms are too often dismissed as too diffuse and speculative to be recognized as actual, real-world injury.
Causation
A second limitation is causation. Negligence law recognizes two distinct types of causation: factual (or “but for”) cause and proximate cause. The first is more straightforward while the latter analysis raises greater uncertainty.
But-for cause requires that the victim shows that the injury would not have occurred “but for” the defendant’s conduct. This limitation makes common sense in that one should not be held responsible for harms one did not actually commit. It is also known as counterfactual cause, a concept readily familiar to AI explainability researchers. In many cases, the mere use of an AI-based system will suffice to prove factual causation. For example, when a fully autonomous vehicle crashes into a highway barrier, there will be little doubt that the autonomous driving has caused the crash. More difficult questions of factual cause can arise if the AI developer claims that additional safety measures could not have prevented the injury, or when there are multiple concurrent causes raising questions of apportionment of fault.
By contrast, the proximate cause inquiry excludes liability when the causal connection between conduct and injury is too remote or unforeseeable to justify holding the defendant responsible. Examples that can cut the causal chain include an intervening event (such as a third-party act or force of nature), an unexpected category of risk, or even the sufficient passage of time or space.
In the AI context, AI developers may argue that downstream uses are unforeseeable, particularly when users enter malicious prompts that hijack the intended purpose of the AI system. But hijackers have sometimes been held to be a reasonably foreseeable vector of attack. Similar questions and uncertainties arise when, for example, a treating physician commits an accidental error while relying on an AI-based diagnostic tool. A stronger argument of unforeseeability exists when the AI model is retrained by third parties and exhibits substantially different behavior than the original AI model.
Much less persuasive is the argument that the AI model itself is an intervening actor that severs proximate cause. Even if the AI system’s behavior truly were so emergent and unpredictable that the AI developer could not foresee the specific harms it would cause, the AI developer likely remains directly responsible for sending out a volatile system without proper controls. The precise manner of injury is unimportant so long as the general nature of the harm should have been foreseen.
Duty of Care
Negligence law applies only when the defendant owes a duty of care to the plaintiff. The modern judicial trend is to define the duty of care very broadly as a general obligation not to create unreasonable risks to others. In addition, special affirmative duties can be created based on a statute, special relationship, contribution to the risk of injury, or other assumption of duty of affirmative care. Accordingly, the duty element typically goes unchallenged and is a non-issue in most negligence cases.
Occasionally, courts will state that an actor owes “no duty” of care toward the victim, which then bars a finding of negligence. As examples, some courts have stated that landowners owe no duty toward trespassers or that a passerby owes no affirmative duty to rescue a stranger. Another vexing example is that an actor owes no duty to prevent third parties from committing harm, except in particular circumstances such as a custodial relationship or when the actor actively enhanced the danger. Some courts have also used the concept of “assumption of risk” to narrow or eliminate the defendant’s duty toward a person who voluntarily consents to a risky activity and is then injured. If there is no duty of care, there can be no breach of a nonexistent duty. A no-duty determination reflects a blanket judgment that an entire category of claims lies outside the realm of negligence law.
Because many AI services are offered for free to the public, there may be downstream uses that injure distant victims who were unforeseeable at the time of release. Some courts might accept the argument that AI developers owe no duty of care to those particular victims. Alternatively, other courts might prefer to analyze the foreseeability question through the lens of proximate cause.
Statutory Restrictions
Beyond common law limitations, Congress and state legislatures can enact statutes that stiffen (or ease) barriers to tort recovery. For example, tort reform efforts have erected numerous procedural barriers such as statutes of limitation, statutory preemptions, shield laws, and certificate of merit requirements that narrow the set of tort claims that can be adjudicated in court. Conversely, legislation has also created or endorsed new types of statutory tort claims, barred inflexible defenses that unfairly limit recovery, and devised no-fault compensation schemes that expedite payment to eligible claimants.
One federal statute that is uniquely salient to AI developers is Section 230 of the Communications Decency Act of 1996, which provides an important immunity for online service providers against negligence lawsuits predicated on third-party user-generated content. Section 230 bars tort claims that treat “interactive computer services” as the “publisher” of such third-party content. While at least some AI systems meet the definition of “interactive computer service,” substantial doubts are being raised whether Section 230 will apply, because AI outputs are generated by the AI system itself and not by third-party users. Moreover, a growing number of courts has recently narrowed Section 230’s scope to permit tort claims based on content-neutral product designs such as addictive features or algorithmic functions.
Because statutory law depends on individual statutes, Congress and state legislatures are free to enact, amend, or repeal AI-specific statutes that push AI policy in one direction or the other.
Alternate Tort Frameworks
The negligence-based approach stands in contrast to other important tort frameworks such as strict liability and products liability in that it focuses on assigning fault to human conduct. At times, strict liability has been touted as a more efficient accountability solution because it streamlines compensation to victims, while also simplifying the “black box” problem of information asymmetries. In theory, where one party has better information on how to reduce harm, a strict liability regime forces that party to use that information or else absorb (or internalize) the costs of not doing so. But if that assumption of superior knowledge fails, and AI developers do not actually know how to avoid causing harms in a cost-efficient way, then strict liability serves only as a deterrent penalty without the information-forcing benefits. To be sure, for critics who worry that AI is not ready for public release, strict liability should remain the preferred policy choice.
More generally in practice, however, courts have backtracked on strict liability doctrines and reverted to negligence-based rules, in part because the latter are viewed as producing fairer outcomes. Products liability, for example, was originally intended to be “strict” in the true sense of expediting compensation to victims without consideration of manufacturer fault. Yet, today, most of products liability law is governed by notions of reasonableness that echo the contours of a negligence claim. Some legacy differences linger, mainly due to the legal fiction that a product can be found defective—that is, faulty—independent of the persons who manufactured and distributed it. Perhaps that fiction remains useful in traditional manufacturing cases where the product is final once released, but the risk in AI cases is that it emboldens AI makers to release their creations to generative user communities and disclaim ownership or responsibility of what happens next. Furthermore, there are lingering questions whether AI qualifies as a “product” when it is continually managed and serviced behind the scenes in real time by teams of people.
Looking Ahead
What should AI developers expect to see under a more robust negligence-based AI liability regime? There are two possible paths, and both would likely intersect heavily with issues of insurance policy.
The first path would continue to classify AI developers as ordinary employees. Employers share liability for negligent acts committed by their employees, as long as those acts occur within the scope of employment. In that event, employers would have strong incentives to obtain liability insurance policies and to defend their employees against legal claims. A potential complication is whether AI companies could disclaim responsibility by classifying substantial portions of AI workers—such as those compiling and cleaning large datasets—as independent contractors rather than as employees. The well-established practice of code reuse, and the fluid boundaries between proprietary code and open-source code, could make it appealing to engage in liability arbitrage by outsourcing as much AI work as possible. How to distribute accountability throughout the AI supply chain will echo similar questions now being raised about the software supply chain.
The second path takes the tack of treating AI developers as professionals like physicians and attorneys. In this regime, each AI professional would likely need to obtain their own individual or group malpractice insurance policies—an insurance product that does not yet exist and that would need to be priced experimentally. Separately, many commentators believe that a licensure bar is an essential prerequisite to professional status. I do not necessarily share that view, but I recognize that occupational licensing is an effective way to screen out uncredentialed workers in a field. To the extent that casual creation of shoddy or malicious AI models by “script kiddies” is socially undesirable, one possible regulatory response is to restrict the practice of AI to licensed professionals. Understandably, a licensure requirement that constricts the labor pool raises policy concerns of global competition in AI. To mitigate those concerns, some form of diploma privilege could be used to facilitate admission of those who are already in the workforce.
Ultimately, AI accountability and AI safety are tied intimately to the quality of the workforce that oversees its constitutive parts. Yet AI is a field that perhaps uniquely seeks to obscure its human elements in order to magnify its technical wizardry. The virtue of the negligence-based approach is that it centers legal scrutiny back on the conduct of the people who build and hype the technology. To be sure, negligence is limited in key ways and should not be viewed as a complete answer to AI governance. But fault should be the default and the starting point from which all conversations about AI accountability and AI safety begin.
– Bryan H. Choi is an Associate Professor of Law and Computer Science & Engineering at the Ohio State University. His scholarship focuses on software safety, the challenges to constructing a workable software liability regime, and data privacy. Published courtesy of Lawfare.